An explosion of open source AI libraries
The open source community has a long history of powering innovation in software, including in software development practices. This will continue in 2019 as the open source community leads the way in adopting AI to improve software development and maintenance practices. The open source community will be both the source and beneficiary of AI-driven advances ranging from intelligent coding assistants to automated code generation to analytics to refactoring.
Corporations will continue to open source their AI libraries as they realise both the inherent advantages in open source and that their competitive advantage isn't in the reasoning engines but rather in the data and learning. This will result in an explosion of open source AI libraries in 2019. Expect more projects, more foundations, and more events until an eventually winnowing to the fittest in 2020 and beyond.
Open source acquisitions and mergers amounted to approximately $55B in 2018. In 2019, we expect to see some big names involved in similar deals as consolidation and competitive positioning take hold. In particular, key open source infrastructure players will be drawn to align more closely with corporate interests, strengthening open source as strategic play but potentially limiting independence and choice.
Democratising access to large training data will level the playing field
Because many of the models we rely on, including deep learning and reinforcement learning are data hungry, the anticipated winners in the field of AI have been huge companies or countries with access to massive amounts of data.
But services for generating labelled datasets are beginning to use machine learning tools to help their human workers scale and improve their accuracy. And in certain domains, new tools like generative adversarial networks (GAN) and simulation platforms are able to provide realistic synthetic data, which can be used to train machine learning models.
Finally, a new crop of secure and privacy-preserving technologies that facilitate sharing of data across organisations are helping companies take advantage of data they didn’t generate. Together, these developments will help smaller organisations compete using machine learning and AI.
Ben Lorica, chief data scientist at O'Reilly Media, Inc. and programme director of the Strata Data Conference and the Artificial Intelligence Conference
Machine learning potential will run into unpleasant realities without high-quality data.
Investors and the tech press are all abuzz about machine learning, but those neural networks are only as good as the training data from which they learn. High-quality datasets—typically the bigger the better—yield more accurate models. As ML initiatives scale, we expect that the pain of cleansing and preparing high-quality data for ML models will become more apparent in 2019. Data preparation is still widely regarded as the biggest bottleneck in any data project, which means that data scientists often spend more time preparing data than actually building and tuning machine learning systems. In order for ML to make an impact at scale, organisations will need to first accelerate their data preparation processes.
Joe HellersteinTrifacta
, co-founder & CSO atAI enables predictability
As we move into 2019, organisations will deploy more technology that enables predictive insights into IT infrastructure. Right now, most IT managers are taking a rearview mirror approach when reacting to unplanned downtime caused by interruptions related to software or hardware error, component failure or something even more catastrophic in the data centre.
Incorporating predictive technologies will enable proactive monitoring for downtime and faults so IT managers can take preventative action before a disruption ever occurs. Being more prescriptive can lead to fewer disruptions and less downtime in operations.
More frequently, AI is enabling predictability and will play a key role in data protection in 2019 and in the future. As businesses are continuing to adopt more complex IT environments, such as hyperconverged infrastructures and other modern workloads, data protection will also need to adapt. AI consistently learns from the system as these dynamic IT environments adapt and change.
Alex Sakaguchi at Veritas
Legacy to cloud: hybrid, multi-location architecture will become the norm
As the cloud initiative progresses with more and more data migrating to the cloud, the center-of-gravity will shift. The balance will tip towards platforms where the data is spread across both cloud and on-premises data sources.
Similarly, integration of the data will transition to a multi-location architecture. Unlike traditional data integration technologies, data virtualisation was designed from the beginning to provide data location transparency, data abstraction, and integrated security across multiple locations, which makes it a perfect fit for these scenarios. Therefore, it will take an increasingly important role in hybrid architectures next year.
Alberto Pan, chief technical officer at Denodo
Intelligent tech to adapt with users
Artificial intelligence has been gathering pace and crucially moving into more practical day to day functions. AI and machine learning is now bringing us to the point where apps are evolving into something bespoke to the individual user. Apps like Spotify can already learn your musical preference and make suggestions based on your previous interaction. This is set to extend to a wide range of apps in everything from travel to cooking and nights out.
In 2019, apps will take data from users’ behaviour and make intuitive changes to how the app operates, improving the user experience. We are going to see more apps effectively learn the preference of the end user, collecting data in real time and adapting the more a person interacts with it.
Chris Costello, director at Sync
Cloud complexity to grow further
Enterprise cloud environments became more complex in 2018, with web and mobile transactions now crossing an average of 35 different technology systems, compared to 22 five years ago. This complexity will grow over the next 12 months, with 53% of CIOs set to deploy more technologies into their cloud environments.
As a result, we’ll see IT teams accept that the old way of IT operations cannot cope with the ever-growing amount of technologies, systems and their dependencies. In 2019, we’ll see cloud operations be transformed by the adoption of artificial intelligence to help IT teams understand and manage this complexity, and also lay the foundations to begin automating cloud operations.
Michael Allen, VP and EMEA CTO at Dynatrace
Moral foundations as important as value
In 2019, the value statement of every vendor that builds AI systems should focus on both the value they wish to create and the underlying moral foundation of their service. How they collect data, with whom they share that data, and what they end up doing with that data will increasingly need a litmus test for what is acceptable and not.
That litmus test needs to be part of the culture of the vendor – it needs to come from the inside out. While this will feel too ‘touchy-feely’ and constraining to some vendors, it is absolutely necessary for long-term business viability to establish trust credibly across their user communities. Without transparency, there is no trust. Without trust, there is no data. Without data, there is no AI.
Ojas Rege, chief strategy officer at MobileIron
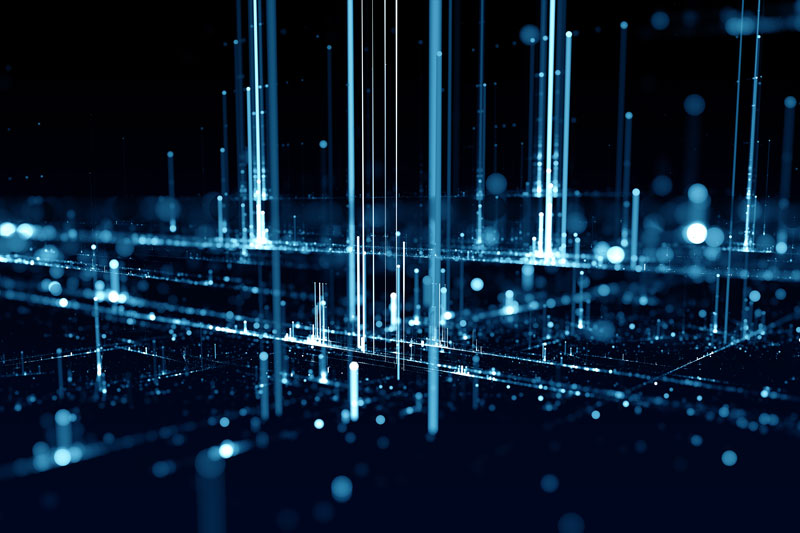
The fragmented customer experience will come to a breaking point
Artificial intelligence is changing the entire business landscape. Gartner predicted that in 2018, AI will create more jobs than it eliminates, and in 2020 it will become a positive net job motivator, creating half a million new jobs. The link between digital transformation and the customer experience is an imperative – and 2019 will be the breaking point.
As companies implement new AI-driven technologies such as chatbots and voice search, they neglect to think about the impact to the customer experience. Customers will become increasingly frustrated with the lack of human interaction and a fractured customer journey as businesses struggle to tie together channels. Customers expect to seamlessly switch from self-service options on web and mobile to chat, email or phone.
Businesses should take a step back, plan customer-centric technology implementations and consider how it ties into the whole customer journey. To win, they must offer customers the right mix of new technology and traditional service. This includes maintaining the human touch by making it easy to access the best person for more complex issues.
Kris McKenzie, senior vice president and general manager for EMEA at Calabrio
AI to go from supporting role to full-fledged assistant
2018 has been a monumental year for AI – especially in the customer service and support sector. The capabilities of chatbots are maturing at a rapid pace and are helping companies deliver always-on, fast and personalised service for their customers.
But AI has really only scratched the surface of what is possible. 2019 will be the year we see AI move from a position of augmenting an experience to becoming a full-fledged assistant. Where today AI-powered chatbots and VCAs are reactionary, we’ll see the technology grow to provide proactive assistance.
Chatbots will evolve from sitting on the sidelines waiting to be asked a question, to being able to anticipate the needs of customers and proactively offer them timely, tailored recommendations based on known information like location, where they are currently in the journey, and past engagements.
Ryan Lester, director of customer engagement technologies at LogMeIn
Machine learning to shift to artificial intelligence
2019 will be the year that machine learning begins the shift to artificial intelligence through the use of complex simulations of biological neurons instead of simple mathematical ones. Machine learning models currently use simplified mathematical models of neurons.
But with specialised hardware, better neuron simulations will lead to the next generation of machine learning, the simulation of biological brains. We can see this in specialised hardware such as the SpiNNaker project, Brainchip's Akida, and Blue Brain's neuromorphic cortical columns. While AI is not real yet, we’re starting to see early evidence of the shift.
Ray Watson, VP of Global Technology at Masergy
AI to meld with DevOps
Cloud migration, increasingly critical big data systems, and spiralling costs and complexity mean that 2019 has to be one of change and renewal. The industry is racing towards new ways of using cloud services to spin up and down a more agile big data stack, and indeed, hybrid cloud models are emerging that take into account internal and external service delivery so that enterprises receive a really customised, cost efficient operation.
If we accept this premise then we’d expect to see AIOps melding with DevOps as a top priority for the enterprise as data is funnelled into training and improving AI-oriented application development and delivery.
As an unrelated factor, it’s a widely acknowledged truth that the talent gap within the DevOps and big data space is becoming quite the challenge. 36% of enterprise business and IT decision makers in a recent survey, conducted for Unravel Data by Sapio Research, discovered that one of the biggest pain points cited was talent scarcity at 3%, followed, perhaps inevitably, by the simple fact that it takes too long to get to insight, as 34% of the sample claimed.
Given 2018’s indications, TensorFlow and H2O will be breakout technologies in 2019, and Spark and Kafka will continue to see accelerating growth as the New year progresses. In fact, the ecosystem of tools the DevOps teams need to draw on will only continue to expand.
Enterprises must find a way to stitch the fabric of the data stack together to get fast, usable insights into the operations powering their BI, customer service, and forecasting applications. Efficiency and effectiveness, however the business measures the KPIs, must be tightly controlled else the whole stack will rapidly spiral out of control in 2019. Unravel’s research showed that although 84 per cent of respondents claim that their big data projects usually deliver on expectations, only 17 per cent currently rate the performance of their big data stack as ‘optimal’. This isn’t a state of affairs that the business can allow to continue.
There needs to be a balance of innovation and control, testing and production, efficiency and effectiveness. Finding that balance will require enterprises to take some calculated risks, but this can be done without live production suffering as automation and intelligence supports the DevOps team in their task of making the magic happening.
Kunal Agarwal, CEO, Unravel Data
Natural language processing to enter the AI scene
If you thought we couldn’t possibly add more technologies to the AI roster than we did in 2018, then think again. In 2019, we’ll see more technologies embraced under the AI umbrella; the most likely candidate will be Natural Language Processing (NLP).
It’s widely accepted as part of AI but has yet to prove its actual AI-ness. NLP helps computers read and respond by simulating the human ability to understand the everyday language that people use to communicate.
There’s huge potential for NLP and as a result there’s a lot of investment into R&D within academia, as well as public and private sectors; Google’s Bert and Facebook’s Py Text are two of the leading developments in this area. Currently Bert or Bert in combination with other technologies are the best performing in NLP and are closing fast on a computer’s ability to read and understand as well as humans, with only a 3% difference.
NLP technologies are already having a massive effect on the industry, creating an explosion of start-ups in all major industrial sectors that all have the aim of automating tasks within business related to language. As we move through 2019, NLP will have enormous implications for the bottom lines of many companies.
Dr Peter Bebbington PhD, CTO and director at Brainpool.Ai
Businesses to change their data strategies
In 2019 we can expect to see AI crossing new frontiers - we are only just beginning to explore applications of AI in healthcare, for example, where new technology has the exciting potential to help prevent, detect and treat a wide range of diseases.
But the industry as a whole has its work cut out for it, if it wants to curb some of the uglier sides to advances in AI. I am not just talking about data misuse, but technical limitations as well. The main issue which will derail the development of AI if not dealt with effectively, is the issue of ‘bad data’ - incomplete, unethically-sourced and poor quality data. When it comes to generating revenue from mobile apps and websites, as organisations only see what happens inside their own apps, sites or ecosystem, a reliance on third-party data from questionable sources provides an incomplete view of the mobile user journey.
In essence, when it comes to AI, it’s arguably better to have no data at all than to rely on bad data. Without the full visibility provided by complete mobile user journey data, the results will be ineffective campaigns, wasted ad budget, and poor consumer perception. We’ll see more businesses coming to realise this in the new year, and taking a more strategic step in their data strategies.
Cedric Carbone, CTO at Ogury
Faster calculations mean better results
While on the face of it 2019 will look very similar to 2018, behind the scenes we will see the development of faster calculations made possible by improvements to the algorithms used in AI. Tools such as Google Duplex, the telephone robot, and e-commerce Chat-bots will be the main beneficiary, as the improvements become more noticeable to customers.
Retailers will continue to adopt practical applications of AI as a key aspect of their sales capture and retention strategies. Visual AI will drive recommendations by narrowing, contextualising and improving the search results that consumers see. Brands will also be able to play with the tone of images used in their communications to make them more appealing. Enhanced recommendation engines will enable retailers to more effectively target shoppers who have abandoned an online basket with personalised and timely communications.
We all know that there is a wealth of customer data that brands can draw upon, even in the era of GDPR. With the continued developments in AI and machine learning, businesses will need to tread carefully to avoid intimidating their customers through excessive personalisation. This will be a critical challenge in 2019.
Boril Šopov, head of AI at Clever Monitor
SD-WAN will continue to evolve towards automation in 2019
Migration to the cloud has become a megatrend. This has led to new requirements in terms of securing services and the required infrastructure. In particular, star-shaped WAN topologies with central Internet access must be redesigned with regard to their compatibility with increasing use of cloud services - keyword SD-WAN.
IoT and Industry 4.0 also open up new areas of attack. Companies should increasingly think about device recognition in the network in order to segment out smart devices accordingly.
Even if it is a truism, e-mail remains the primary gateway for malware. Users can now protect themselves much better against this with intelligent email security products.There is still a lot of catching up to do here. Therefore, all necessary security technologies should always be preceded by a well-founded education of the employees.
Companies must develop a comprehensive security awareness programme that addresses the most important security issues. The solutions will continue to evolve towards automation in 2019.