Thought Leaders
An Intelligent, Targeted Approach to Optimising AI Enterprise-Wide
Headline-grabbing buzzwords have drawn considerable hype towards artificial intelligence’s potential in business, but the reality is less sexy. Bob De Caux, VP AI and RPA at IFS explains how data management is key to success
Cool or creepy, the idea of integrating robotics, artificial intelligence, machine and deep learning (ML; DL) into a business has been hugely hyped over the past decade.
Robots replacing workers and a fourth industrial revolution make for sexy headlines. However, the real currency for the success of AI is the slightly less headline-grabbing, but a lot more important, domain of data management. Get this right, and you can transform your business.
Productivity and the AI problem
UK workers’ productivity was lower over the past decade than at any other point throughout the whole of the 20th Century, according to recent findings by the Office of National Statistics.
AI (and the multitude of other acronyms in this tech space) has understandably been positioned as a panacea to businesses’ woes, by recovering an estimated 6.2 billion hours of worker productivity by 2021, and generating $2.9tn for its adopters.
Again, these make for sexy headlines, but where’s the substance? We’ll celebrate the arrival of 2021 in just 18 months; will businesses really be hitting these forecast figures by then?
According to a 2018 survey by McKinsey & Company, only 21% of organisations have embedded AI in several parts of their business; an approach which will be necessary to fully realise the technology’s benefits.
The majority of respondents also revealed they spend less than one-tenth of their digital budgets on AI, painting a reality which is far from the AI-powered, productivity-boosting revolution many analysts (and the media) are hoping for.
“We’ll celebrate the arrival of 2021 in just 18 months; will businesses really be hitting these forecast figures by then?”
This isn’t to say there haven’t been developments in this space – and especially in the UK, which, alongside other European nations such as Germany, has played a leading role in the development of AI and deep-learning technologies.
The field service management sector, for instance, is pretty mature in terms of using AI for fleet optimisation, i.e. choosing the best route for their drivers in order to fulfil all their jobs most efficiently.
The utilities sector has also been using AI and ML models for some time; some energy companies, for example, are using the latter for predictive maintenance, detecting unusual patterns in data they’re receiving from energy assets, and highlighting these to engineers. In manufacturing, meanwhile, AI solutions are enabling organisations to minimise waste, and customise production at scale.
The rate of AI integration, as well as – more importantly – the methods of integration – vary substantially across different markets. While the UK in general is ahead of the AI curve, some sectors continue to lag behind. Areas like field productivity, safety monitoring, and quality control could all be improved and optimised by replacing laborious manual processes with self-learning and automation.
The problem? As with other markets that have yet to embark on an AI journey, it’s a lack of data, and/or a coherent means of consolidating multiple data sources.
The lifeblood of AI
Data is the lifeblood of AI. As much as some of us might like to imagine the machines and technology of the future as cognisant beings, at the moment their ‘thinking’ and ‘actions’ are determined by algorithms, data, rules, models, and transactions.
The ‘learning’ which informs output and responses needs data to be successful – more often than not overseen by humans. Many companies operating in the construction industry, for example, suffer from a lack of comprehensive datasets, which are needed to train and maintain AI.
Add to this the growth of the IoT, which is already spreading its millions of end-pointed tentacles into a business, and even early adopters of AI solutions now find themselves in the position of needing to effectively integrate a continuous stream of real-time data with their traditional data sources.
Construction is a hugely diverse sector, but businesses (encompassing housing, irrigation, municipal engineering, petrochemicals etc) share common characteristics: they have engineers and designers, need to hire equipment, manage offsite manufacturing divisions, handle logistics, supply chains, as well as internal business processes from finance and HR to CRM.
“Even early adopters of AI solutions now find themselves in the position of needing to effectively integrate a continuous stream of real-time data with their traditional data sources.”
All of these generate significant volumes of data, which if collated, analysed and easily accessed by all employees within a company, can help optimise processes, reduce waste and modernise the construction industry.
AI and ML, embedded in enterprise resource planning and asset and service management tools will be crucial. Harnessing business-wide data, these solutions will ensure firms can better evaluate risk, manage and coordinate supply chains, and orchestrate robotics, paving the way for approaches like modular housing construction: a growing market estimated to be worth $52100m by 2025.
Implementing AI is not as simple as finding an off-the-shelf solution that will instantly transform your business though, particularly as a proportion of these solutions will be peddled as advanced, proactive AI.
The reality? Many offer little more than basic analytics. AI is not a magic wand that can be waved over a business to create instant, transformational change. Neither is it a sticking plaster that can be layered onto existing technology and processes in the hope that it’ll make everything better.
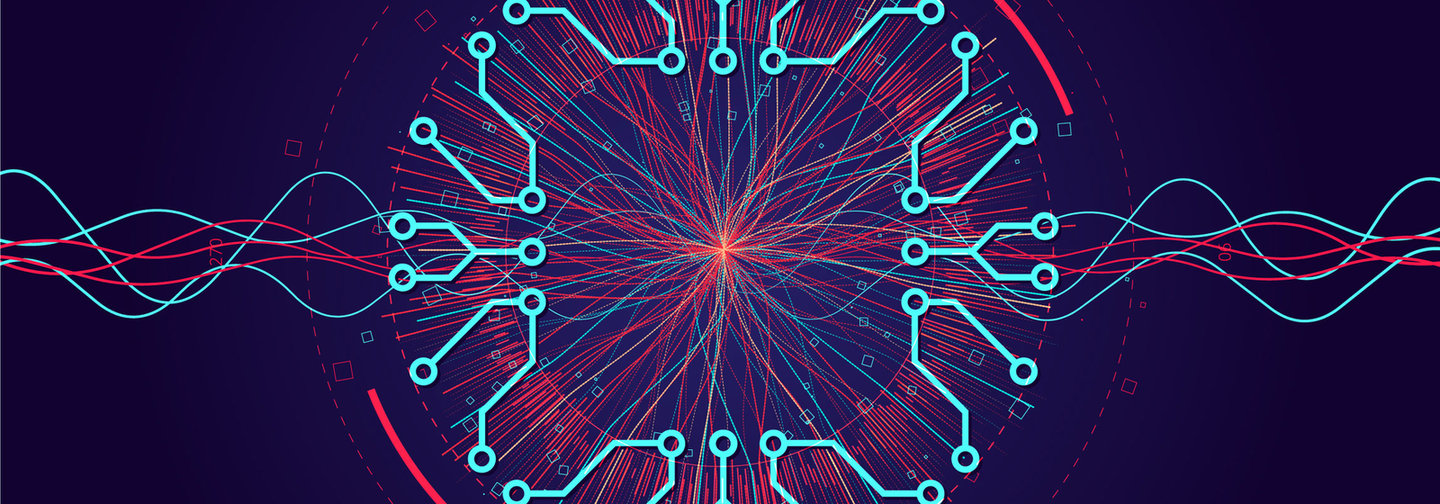
Weaving the fabric of business strategy
Any company looking to implement or develop AI within its business – be it construction, insurance, healthcare, manufacturing, automotive, broadcast, retail, aerospace (the list is endless) – should do so as part of a business-wide, top-down strategic process.
Rolling out AI in one area to solve one problem is relatively straightforward but the value of this is meagre. AI must be woven into the fabric of a business’s strategy, extended to multiple business processes, and involve a holistic view of data and a re-skilling/up-skilling of your workforce.
Siloed data must be unified and spreadsheets should be scrapped. Despite what many of us like to think, I’ll bet spreadsheets will be found not only lurking in, but leading fundamental processes within most companies today.
“Despite what many of us like to think, I’ll bet spreadsheets will be found not only lurking in, but leading fundamental processes within most companies today.”
Sourcing, logging and analysing disparate data sets depends on communication between every sector within a business, and the use of ERP platforms that create a collaborative, accessible environment.
Implementing targeted AI solutions as part of a wider strategy has already resulted in the democratisation of data for many businesses, allowing them to harness and learn from data from a growing number of sources.
These include many in the manufacturing sector – which in general is further along its (collective!) AI roadmap than a sector like construction – such as Japan’s NEC, which has integrated voice-activated solution in its order picking process, while Amazon is deploying robots to pick and pack customer orders in its warehouses.
A view for the future
Call it industry 4.0, the robotic revolution, or human augmentation – AI will be used by the vast majority of businesses in some form in the near future. Its efficacy and success will be reliant on its users gaining a holistic, centralised view of their data assets.
The amateur dabbling in commoditised, open-source AI and commercial off-the-shelf-type solutions we’re seeing today must evolve into the integration of bespoke AI tools which allow users to build their own data models and tailor the AI to the specificities of their business.
It’s this kind of approach that will deliver business efficacy and success long after the hype has died, extracting real value from intelligence, for the long-term.