Product Focus
From Emotion to Sale: Behavioural Signals’ Voice-Reading AI
Artificial intelligence that can predict if you’re going to buy from the emotion in your voice may sound like a technology on the horizon, but it’s already a real product. Robert Scammell speaks to Rana Gujral, CEO of Behavioural Signals, about the company’s technology
Imagine knowing a person’s intention to buy a product with more than 80% accuracy, solely from the emotion in their voice. That is the promise of Behavioral Signals, an artificial intelligence (AI) startup based in Los Angeles, US.
The firm’s core product is its ‘emotion engine’, which uses machine learning to “deduce emotion from voice data” and turn it into usable information.
“Most humans aren’t very good at understanding emotions, even though we’re supposed to [be],” says Behavioral Signals CEO, Rana Gujral. “We miss those certain cues.”
The two and a half-year-old firm seeks to spot these missed verbal cues, providing businesses with a tool that can provide an indication of a person’s intent.
“We focus a lot on very specific binary KPIs [key performance indicators],” Gujral told Verdict at AI Everything, a conference in Dubai, UAE, that attracted thousands of business delegates and AI experts in May.
That means answering a binary question, such as whether a person is going to pay their debt, during a phone conversation. In turn, a business can then reach better outcomes more quickly.
The “Tensorflow of emotion engine”
In the case of a debt collector, a call centre agent can change their approach when they know – from the voice data – that the customer is not very likely to pay, such as offering different incentives.
It’s not just debt collectors that can use Behavioral Signals’ AI tech. Its platform is “vertical agnostic” and targets sectors including finance, retail and education, among others. Its tech can also be integrated into virtual assistants and robots.
Behavioral Signals, which was co-founded by Shri Narayanan, a leading professor of behavioural science, is more of a behind-the-scenes firm, selling its emotion engine to businesses for their own business needs.
“We think of ourselves as the Tensorflow of emotion engine,” says Gujral, who describes his company's offering as “emotion as-a-service”.
“Any place where there’s a human to machine interaction, we have the ability to have the machine be more emotionally aware, and be more in tune with how the human is feeling at a certain point,” he says.
How does Behavioral Signals work?
Gujral says that the company’s emotion technology can be broken down into three parts.
The first is speech synthesis: how fast a person is speaking, speaker overlap, ratios, and who is speaking when.
“The engine can deduce in real-time who is speaking, when certain words are spoken and how fast the person is speaking and who is speaking most and who is speaking least etc.”
The second aspect is linguistics. This focuses on the “what” is being said.
The third is how words are being spoken – intonations and tonality. “It’s the emotion and the behavioural portion behind the words that are being used.”
Behavioral Signals’ algorithms crunch all of these factors and provide an answer to the binary question.
And it is able to confirm its 80% + level of accuracy by running it on historic call centre datasets where the outcome is already known.
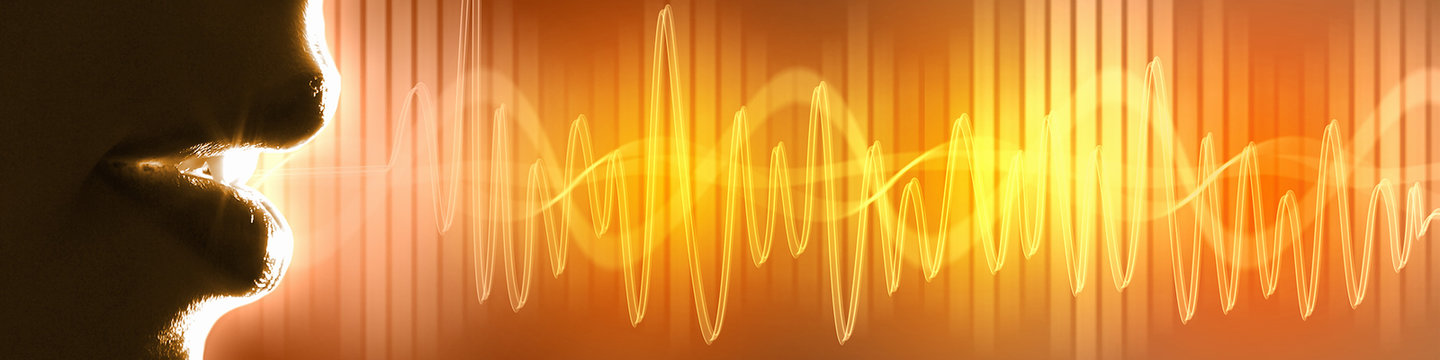
With great power comes great responsibility
However, as with any technology, Behavioral Signals’ emotion engine comes with social responsibility.
“You could misuse this technology quite a bit,” says Gujral. He explains how one “large company” approached Behavioral Signals for a very specific use case for “social good”.
It involved using Behavioral Signals’ emotion engine to detect the abuse of children in the home or elderly people in care homes.
“You could misuse this technology quite a bit.”
“So they want to build this device that is in your home, is always listening – is listening to everything. And it’s passing out specific signals of distress and duress and abuse. And, based on that, certain actions will be taken: alerts, [the] authorities might be called, etc.”
Gujral describes it as a “phenomenal use case”, but as with many good intentions, it comes fraught with problems.
As well as the privacy infringements, it also opens up the possibility of predicting crimes before they have been committed based on intent, reminiscent of the ‘Minority Report’.
“You could apply that into sneaking into people’s lives, police can monitor you, big government can get into their homes and start to understand and take action based on your intent.”
Training data is key
Key to any AI program is an abundance of data to train the machine learning algorithm. According to its website, Behavioral Signals has analysed 1,219,008 conversations. But more data is needed to keep improving the engine, which it gets working with “qualified partners”.
“There’s a fine balance. We’re pushing the needle of the technology, but there’s also a lot of concern,” he says, citing privacy laws such as GDPR.
“Those things sometimes make things harder. I think it’s for the good, I think those measures are supposed to be in place for the right reasons. But that also makes our job harder.”
“Now, you have the ability to take the best calls and worse calls, understand why they’re good, why they’re bad.”
For companies auditing the performance of their call centres, the tool can take away the heavy lifting performed by humans.
At the moment, the process involves picking a random set of calls from a random set of departments. Someone listens through, looking out for violations.
But using the emotion engine, “based on emotion signs”, the calls can be categorised into good and bad calls, or red calls and green calls.
“Now, you have the ability to take the best calls and worse calls, understand why they’re good, why they’re bad,” explains Gujral. “You can take the red calls per department, you can take the red calls per agent, you can find an agent with the worst calls, you can find the agent with the best calls – it’s a much better audit process.”
“Nobody has anything like this”
A lot of Behavioral’s clients are behind-the-scenes and therefore their names must be kept confidential. But he can say that they are piloting a product with Disney, which is looking to use it for “other interesting purposes outside of call centres”.
But does Behavioral Signals use their own tech on potential customers to predict their buying intent?
In short – no.
“What we would do is give the emotion engine to the client and say ‘hey, take the calls from the past, you know the outcome already,” says Gujral.
“Apply the engine, the engine’s going to predict the outcome. You know the outcome – measure it. You come back to us and tell us how accurate that is. When that happens, the sale is done. Nobody has anything like this.
“We now have a tool that can predict intent and behaviour, it’s a game changer.”